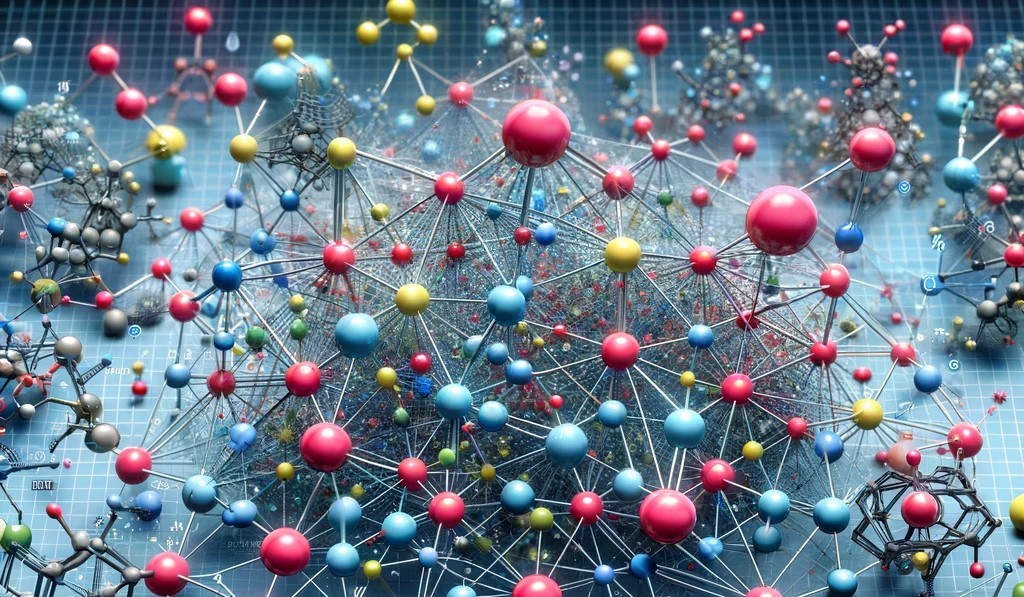
For decades, pharmaceutical companies have relied on high-throughput screening (HTS) as the first step in the drug discovery process. After an initial screening of thousands of compounds, scientists select a smaller list of candidate drugs that is then used for further downstream testing. A major limitation to HTS, however, is the need to synthesize all compounds used in the screen—the compounds need to physically exist to be tested. This significantly limits the number of compounds that can be tested, hindering the discovery of new drugs.
What if we could test compounds even before they are synthesized?
This may be possible with computational approaches utilizing artificial intelligence (AI). Computational AI methods allow researchers to evaluate a vast library of virtual compounds without the physical existence of compounds at the initial screening stage. The development of AI methods is challenging, however, as they require extensive target-specific training data and high-quality X-ray crystal structures. Due to these limitations, previous attempts using AI have not been hugely successful in accurately predicting bioactive compounds. However, a new computational screening system, called AtomNet, has finally overcome these challenges.
AtomNet is like a digital map where each point represents an atom, and lines between them show how close they are to each other. It looks at groups of atoms that are part of bigger molecules (like potential drugs and their target proteins) but only considers those close enough to potentially interact. This model learns from many examples to make predictions about how well a potential drug might work, how it fits with its target protein, and the strength of their interaction, using a special training process to improve its accuracy. Importantly, this model does not rely on target-specific training data, structural data, or manual cherry-picking.
A recent publication in Scientific Reports demonstrated the predictive power of AtomNet. The authors used this model to identify candidate drugs, or “hits”, for 318 targets of interest. They collaborated with hundreds of academic and screening labs around the world to physically validate these hits. Remarkably, AtomNet successfully identified structurally novel hits for 73% of the evaluated targets. This is significantly higher than the success rate of 50% for traditional HTS.
Besides the high success rate, AtomNet was also able to identify novel, drug-like scaffolds that are not merely slight modifications of known bioactive compounds. For example, the model identified viable candidates for challenging targets involved in protein-protein interactions and those without known drug-like inhibitors. This opens up a vast new world of possibilities for drug discovery.
The success of AtomNet suggests that we may have reached a turning point in drug discovery. AI may soon replace HTS as the primary method for initial screening. This shift towards computation methods could lead to more efficient, cost-effective, and expansive search for novel therapeutic drugs. As AI models continue to evolve and improve, they may redefine the boundaries of pharmaceutical research, ultimately benefiting patients suffering from previously untreatable diseases.
Learn more about our solutions for Small Molecular Drug Discovery.
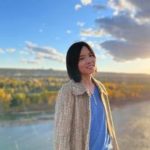
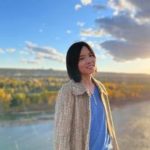
Latest posts by Johanna Lee (see all)
- Can AI Replace High-Throughput Screens for Drug Discovery? - April 30, 2024
- How Avian Influenza Crosses Species - February 22, 2024
- Macrophages: The Unsung Heroes of Immune Response and Biologic Drug Development - December 28, 2023